News
RESPONSIBLE AI INTEGRATION DEMANDS A LAYERED APPROACH - VUKOSI SAMBO
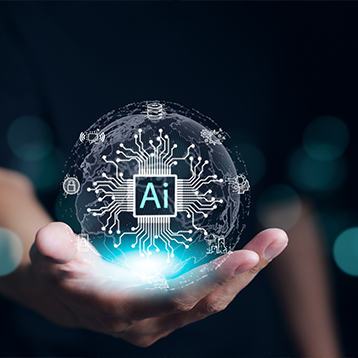
The adoption and integration of artificial intelligence (AI) has rapidly spread across our global economy encompassing pretty much every sector and industry. The recent developments in Large Language Models like ChatGPT has caused an even more exponential rise in use of AI, capturing the imagination of people around the world. Thanks to their broader utility- almost anyone can use these tools to communicate and create solutions with ease.
Alongside the recognition of AI’s benefits, there is a growing consensus that we need to tackle the risks and potential harms these technologies breed like bias and discrimination. Implications of AI can be far reaching and extend beyond the boardrooms of a business, but to wider societal impact.
A multitude of AI ethics principles have been proposed to tackle these risks, but corporate and organisational processes and protocol to ensure socially responsible AI development are largely lacking. A comprehensive governance model is required to bridge the gap between principle and practice by AI practitioners within organisations.
I propose a layered approach with useful recommendations that organisations should follow to achieve integration between theory and practice. This naturally shines a spotlight on the responsibility of the organisational stakeholders to drive collaboration in this AI journey.
To succeed in this AI endeavour, I propose four layers – i.e. societal, industry, organisational, and internal AI systems. Let me outline the interconnectedness of the layers to each other when held by a harmonious and robust management system.
Layer 1: Societal environment - AI for good
This layer demonstrates the highest level of maturity in the AI governance framework. It is all about demonstrating the AI system’s role in driving sustainable social and environmental gains beyond direct economic benefits to the organisation.
This represents the qualitative role that the organisation accepts and embraces as a force of social good, and as a corporate citizen. Often referred to as ‘AI for good’, this can be specific to either the sector or wider societal level.
These efforts align with a primary United Nations Sustainable Development Goal (SDG) for the healthcare sector and a foremost target for the AfroCentric Group – Good Health and Wellbeing.
Our ambitions at AfroCentric specifically address universal health coverage, including financial risk protection, access to quality essential healthcare services and access to safe, effective, quality and affordable essential medicines and vaccines for all.
For example, our AI-driven fraud, waste, and abuse (FWA) system recovers over R150 million annually in our financial risk protection efforts. It is at this layer that the AI ethical considerations must be formulated. There are two important factors to consider - how understandable and ethical they are. Understandability means that the AI should be transparent, fair, and keep your privacy and security in mind. It should also be clear why it is making certain decisions. Ethical considerations include making sure the AI is inclusive and reliable, and that it is held accountable for its actions. Safety is also a top priority.
Layer 2: Industry environment – Stakeholder inclusivity
This layer focuses on the role of AI systems to improve performance of the sector in a way that benefits all stakeholders. This usually manifests itself through organisational ecosystem and platform models together with ecosystem partners and even competitors. Success at this layer greatly enables the societal environment layer through removing barriers to participation.
Layer 3: Organisational layer - Strategic alignment
Organisation value and strategic alignment to the legal and ethical framework is what this layer is all about. When crafted well, this brings to life the core organisational values through a practical execution framework of organisational capabilities.
The greater the alignment through tangible behavioral integration, the more evident the culture of responsible AI implementation and digital transformation adoption in the organisation will be.
Layer 4: Internal AI System – Execution & operational governance
We all know that the devil is in the detail. This is where the rubber hits the road when it comes to actual implementation of AI technologies into your operations.
This is where you discover if your system is optimising operations, delivering a better customer experience, enhancing product and platform offerings, and better managing risk.
It is best to think of this layer as the operational governance layer. It assists day-to-day AI and data practitioners, and managers embedding the other three layers into tangible processes. These are processes concerned with development, use, and management of AI systems.
The role of robust management systems for layer integration
The key to tying the bow on all these layers is a robust and living management system. This should assist stakeholders responsible for each layer to drive the best outcomes for their layers and the overall framework at large.
You need to develop the ability to extrapolate the intricacies inherent in each layer into a Responsible AI Scorecard to aid compliance reviews of the management system by technical and non-technical stakeholders. This provides insights that help monitor and manage performance of the AI systems, bringing to life the AI governance model for board oversight.
To empower the board and associated sub-committees in this regard, two categories of the Responsible AI scorecard are particularly important:
- Risk and Impact focus: AI system harms and impacts pre-assessment; Algorithm risk assessment; AI system health, safety, and fundamental rights impact assessment; AI system non-discrimination assurance; AI system impact minimization; AI system impact metrics design; AI system impact monitoring design; AI system impact monitoring; and AI system impact health check.
- Compliance focus: Regulatory canvassing; Regulatory risks, constraints, and design parameter analysis; Regulatory design review; Compliance monitoring design; Compliance health check design; Compliance assessment; Compliance monitoring; Compliance health checks.
Other categories of the scorecard should include AI system design, algorithms review, data management operations health checks, transparency & explainability, development, and accountability & ownership.
At AfroCentric, we apply this conceptual framework to design best in class AI and secure data solutions to improved access and health outcomes of our 3.8 million members, yielding well over R4 billion in cost savings for our scheme clients annually. This directly impact cost of care, improving access in line with our core purpose, aiding the sustainability of health system at large, and contributing meaningfully to SDG imperatives.
The question remains: How can AI transform your business? Apply the layers and find out!
Vukosi Sambo is the Executive Head of Data and Insights at AfroCentric Group. He also serves in several data ad technology advisory boards and editorial boards. He is global multi-ward winning data and healthcare executive, and keynote speaker at data and technology conferences.
Back to previous page